The Bird Lending Dapp is a non-custodial digital asset lending and borrowing platform based on the Compound protocol with altered asset pools and the use of the BIRD Score to increase access to additional funds in excess of the current collateralized debt positfions offered by Compound Finance and AAVE.
CDP Explained
The Collateralized Debt Position (CDP) allows a DeFi user to lock their crypto assets (currently only ETH) into the CDP vault and receive a loan against the deposited sum of 66 percent DAI (1 DAI = 1 USD). The user only needs to repay their borrowed debt in Dai in order to unlock and reclaim their locked crypto assets.
Example:
- Lock up 100ETH in the collateral vault
- Borrow up to 66ETH worth of Dai
- Repay borrowed Dai at any point in the future to unlock and reclaim collateral
The problem users are faced with in the current scenario is that 100 ETH is required to receive a loan worth 66 ETH in value. Bird.Money is looking to change that requirement.
Why is the BIRD Score so important?
The BIRD score allows lenders to tailor their financial products to each customer, including varying the collateral requirements for a DeFi loan. However, for lending protocols to decrease collateral requirements while still maintaining safe liquidity levels, the score must accurately reflect whether a borrower is likely to repay their loan. That’s what makes Bird’s methodology so important.
Several projects have set out to build an on-chain credit score, but nearly all of them rely upon a far less accurate method to create the formula for their score: human judgement. To understand what this means, let’s break a credit score down into its two main parts: attributes and weights. Attributes could include things like the age of a wallet or prior loan repayment history. Weights are nothing more than numbers assigned to each of these attributes, that when combined together, create the score. How these weights are set determines the accuracy, and therefore usefulness, of the score in predicting risk. So while the competition is setting their weights based on their intuition, Bird developers will be hard at work building cutting edge statistical models, such as deep learning artificial neural networks, to set the weights used in the BIRD score. Risk is complex and this complexity is better captured by mathematics than human judgement alone.
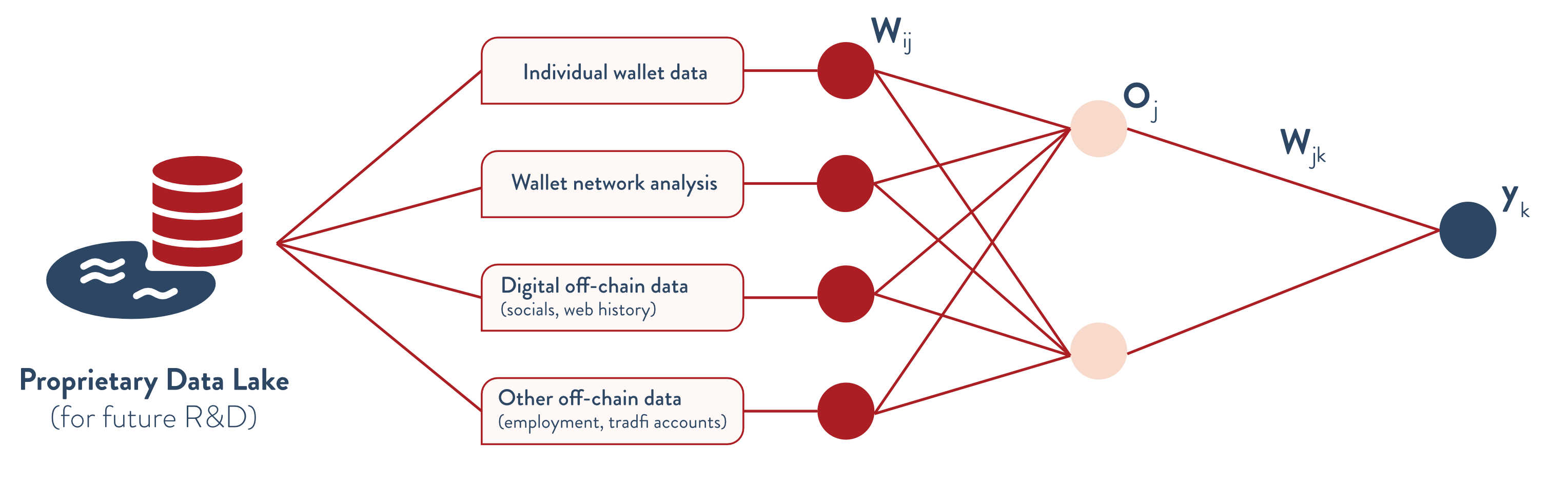